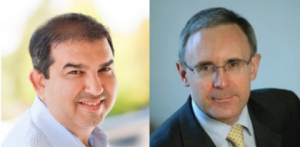
How should companies go about acquiring new customers? How can they make sure that their discount offerings are going to result in acquiring new customers? How can they estimate the total lifetime value of a specific individual customer? How can they make the most of their marketing expenditures? How to predict how much a consumer will spend? These are some of the questions I touched upon during my trip to the Silicon Valley with Jack Voileau when we met with Omer Artun, CEO of AgilOne.
I/ How does AgilOne proceed?
AgilOne is trying to provide marketers with a solution where they can see what customers are doing across every single touch point with the brand. And then from there, AgilOne is setting up a specific set of analytics to understand customers both at a descriptive level and at a predictive level.
For example, descriptive elements may include:
- How tall are you?
- How much did you spend?
- What’s your email click rate?
- Is your email click rate increasing or decreasing?
These are explicit questions. And then these explicit questions are the foundation for the predictive element, which may include:
- How much will you spend on the brand?
- What will the average purchase turn out to be?
- How much will the consumer spend on the brand during his entire lifetime?
II/ Clustering helps to make predictions. That’s key to predict how much a consumer will spend
Humans like to group things together. So they have a clustering solution that clusters things together, based on different schemas, including behavioral schemas. This will show differentiate the consumer base. For example, it may show that:
- This consumer set are discounters
- This consumer set are high value customers
- These customers hop a lot but don’t buy a lot
Another schema may originate from brand type:
- High end brands like Gucci
- Consumer brands like Puma and Addidas
The key here is to help marketers group customers in different ways, so that he/she may differentiate discount sensitive consumers from sport buying consumers, for example.
Following this behavioral clustering, marketers can adapt their marketing accordingly. In addition, they can use this knowledge to predict future consumer behavior. Today, marketers want to predict:
- if a customer will come back and buy
- if they will open an email if a I send them one.
- how much they will / could spend on my products?
- if they are going to go away?
- the life time value of customers, based on what their purchasing history
It’s not about predicting the lifetime value of customers accurately. It’s about sorting different types of customers. For example, a key output may be: “here are my high value customers who have a large wallet. Now I want to target those people because they will spend a lot of money. Every business churns customers, so I need to target customers that will spend a lot of money with my company’s products”
III/ How to reduce marketing expenditures and attract new customers?
Let’s say it costs 1 dollar to reach one customer. We’ll assume that there are 2 customers, who have both completed purchases, at least once.
- Customer A has a 5% probability of coming back and completing another purchase
- Customer B has a 1% probability of coming back and completing another purchase
What most marketers do, is that they offer a 10% discount for everyone. But this is not the best way to proceed, because:
- some customers have a very high chance of coming back and purchasing, regardless of the discount
- other customers have a much lower chance of coming back and completing a purchase, regardless of the discount
Thus, Omer says : don’t do that. Because, let’s assume that it costs $1 to reach a customer.
- Customer A has a 5% chance of buying. With customer A, marketers have to reach out to him 20 times in other for him to complete a purchase. Given that reaching out just one time to a customer cost $1, it costs marketers 20$ (20 X $1) to reach him
- Customer B has a 1% chance of buying. With customer B, marketers have to reach out to him 100 times in other for him to complete a purchase. Given that reaching out just one time to a customer cost $1, it costs marketers 100$ (100 X $1) to reach him
Now the AgilOne suggests that marketers say:
I’ll give a 0$ dollar discount to customer A and a 50$ dollar discount to customer B.
- When I provide the discount of 0$ to customer A, the change of customer A purchasing went from 5% to 5%. As a consequence, the cost of customer acquisition remained at 20$ for customer A
- When I provided the discount to customer B, the chance of customer B purchasing went from 1% to 3%. As a consequence, the cost of acquisition went from 100$ to 83$ ($33 accounts for the cost of reaching out to customer B and $50 accounts for the cost of the discount). In other words, targeting my discount to a relevant customer group helps me to reduce marketing expenditures by $17.
More broadly speaking, by operating like this, and knowing that some customers, like customer A, should not get an offer and other customers, like customer B, should get an offer, I’m able reduce marketing expenditures by 20%, according to Omer.
This summarizes the discussion I had with Omer Artun and Jack Voileau.
Further readings on how to predict how much a consumer will spend
- For another description of AgilOne’s offerings, please refer to their blog, here
- For an interesting article on how to email different segments, please refer here
- For another article on AgilOne, please refer here
- For an article on pitfalls in data-driven marketing, please refer here
- For a wealth of information on predictive marketing, please refer to this blog
- For a simple predictive marketing solution, please refer here
[…] What will the …read more […]
[…] Pour un exposé approfondi sur la Big Data et ses usages futurs, lire cet article. […]
[…] Pour comprendre comment le Big Data peut prévoir la quantité d’argent que va dépenser une personne pour une marque donnée durant sa vie, lire cet article de blog. […]